The Future of Analysts: Orchestrating AI for Strategic Insights
I’ve written a couple of posts examining the significant challenges confronting startups in the AI landscape, particularly those involved in training foundation models like Large Language Models (LLMs). In one post, I highlighted Meta's release of the world's largest "open weights" foundation model—a notable development in an environment where OpenAI, another major player, is grappling with serious financial difficulties. These challenges go beyond just funding; they question the very sustainability of business models for many LLM startups. The market is increasingly tilted in favor of deep-pocketed companies, creating a "winner-take-all" dynamic that makes it exceedingly difficult for smaller players to gain a foothold.
In another post, I delved deeper into OpenAI's financial struggles, noting that their operational costs could reach a staggering $5 billion by 2024. Escalating model training costs, a lack of differentiation and clear product-market fit, and mounting regulatory pressures combine to create significant challenges within the LLM space. This landscape suggests that while the core AI model architecture is critical, it might not be where the most accessible opportunities for innovation lie.
The challenges extend beyond just training LLMs. The market for providing inference endpoints for foundation models and LLMs is equally competitive and challenging. Numerous startups and companies are serving models, resulting in a plethora of capable options and endpoints. This abundance makes differentiation difficult and often turns pricing into a race to the bottom.
Despite these hurdles, I believe the real opportunities are emerging at the application layer, where differentiation is still achievable. Startups that focus on creating unique, industry-specific Generative AI applications—such as in healthcare or finance—can still thrive by leveraging existing models while offering specialized functionality.
The Current Landscape of LLM Utilization
Given these foundational challenges, it's interesting to see companies beginning to use LLMs in a variety of ways. Recent LLM job postings in the U.S. reveal that businesses are harnessing LLMs primarily for text generation and natural language understanding. These two capabilities are central to many current deployments, appearing in approximately one-third of all job listings. This data underscores the fact that companies are heavily focused on tasks that involve creating human-like text and interpreting complex language inputs—two areas where LLMs have proven particularly adept.
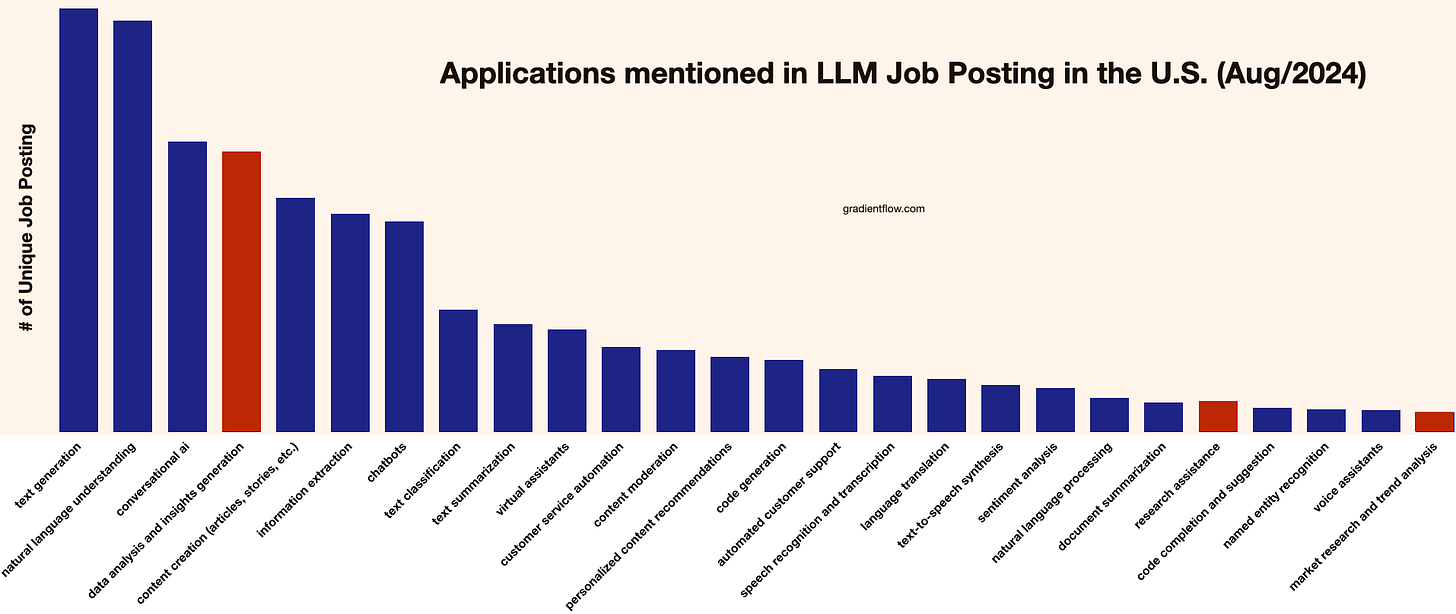
Conversational AI and data analysis are also prominent in these job postings, each appearing in about one-fifth of the listings. This suggests that businesses are not just interested in LLMs for generating content, but also for interacting with customers and extracting insights from large datasets. The practical applications of LLMs extend to content creation, information extraction, and chatbots, all of which are becoming integral parts of how companies operate and engage with users.
Furthermore, there's significant interest in specialized applications such as text classification, summarization, and virtual assistants. These use cases, along with customer service automation and content moderation, suggest companies are exploring ways to streamline operations and improve user experiences through LLM technology. Applications in areas like code generation, speech recognition, and language translation hint at a broadening scope of LLM utilization across various industries.
Analysts in the LLM Era
I'm particularly intrigued by the ways in which LLMs are beginning to influence the role of analysts in organizations. As these models gradually integrate into various workflows, they're changing how information is processed, analyzed, and utilized. This shift is starting to become noticeable in areas like research assistance, where LLMs are beginning to show their value. For instance, these models can assist in analyzing large volumes of research literature, identifying relevant papers, and summarizing key findings. As LLMs continue to advance, they promise to reshape the analyst's role, potentially transforming how we approach research, identify trends, and generate insights across various disciplines.
Additionally, there's a growing interest in how LLMs could streamline the interaction between analysts and data through natural language interfaces. Some tools are now enabling analysts to query databases using plain English, which reduces the need to craft complex SQL/Cypher queries. This could democratize data access, making analysis accessible to a wider range of professionals. LLMs are reshaping the field of data analysis by demonstrating promise in identifying intricate patterns and trends within large datasets. These models are beginning to augment human analysts' capabilities, unlocking new approaches to sentiment analysis and opinion mining, although their practical applications are still being refined.
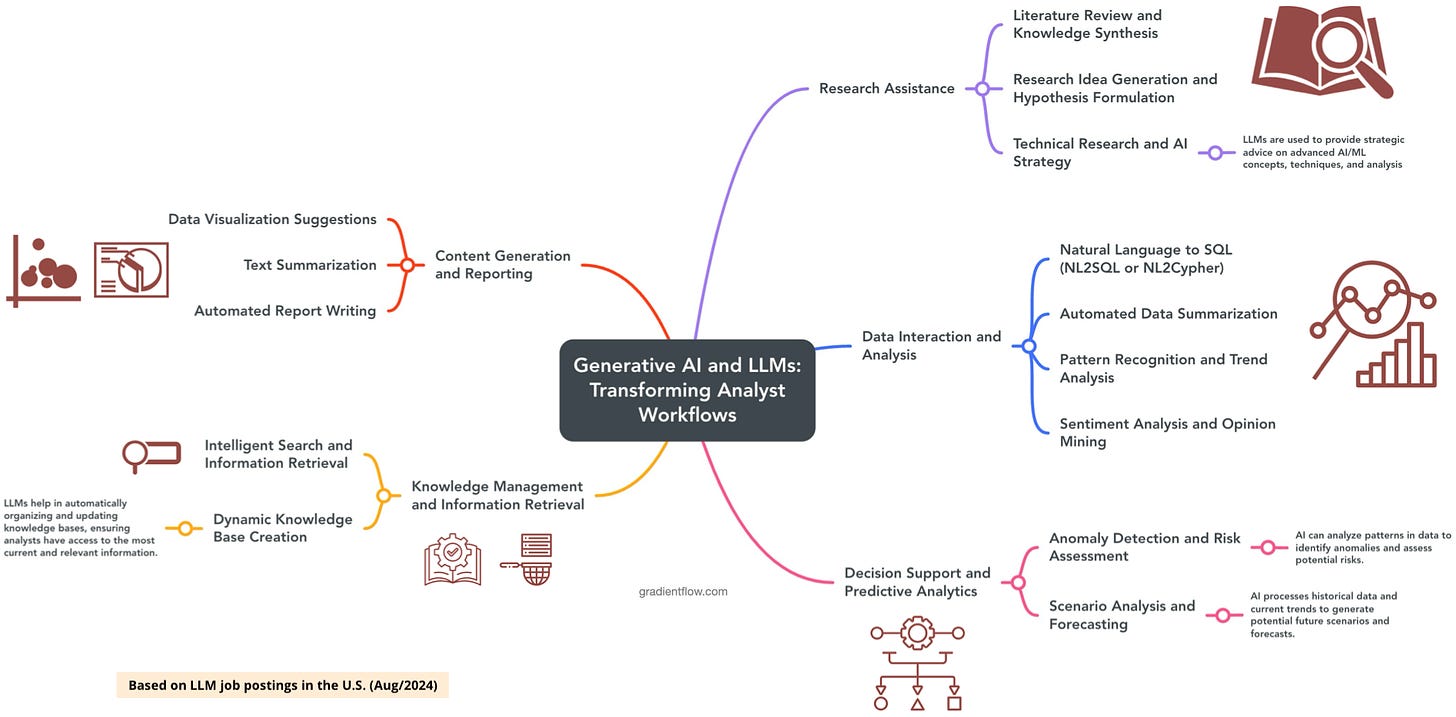
LLMs are also starting to make headway in content generation and reporting. While not yet widespread, some models are capable of generating coherent, data-driven reports, which could free analysts to concentrate on higher-level analysis and strategy. Furthermore, LLMs are beginning to enhance knowledge management by improving search capabilities, understanding context and intent, and helping ensure that analysts have access to the most current and relevant information.
The Analyst of Tomorrow
The future of analysis isn't about replacement; it's about augmentation. Every analyst should be using Generative AI tools today—not just to keep pace, but to redefine their roles. As LLMs advance, analysts will evolve into hybrid professionals, merging deep domain expertise with AI literacy and critical thinking. Anecdotally, I've come across stories of analysts—and even junior lawyers—being able to take on more work thanks to Generative AI, expanding their capacity without sacrificing quality.
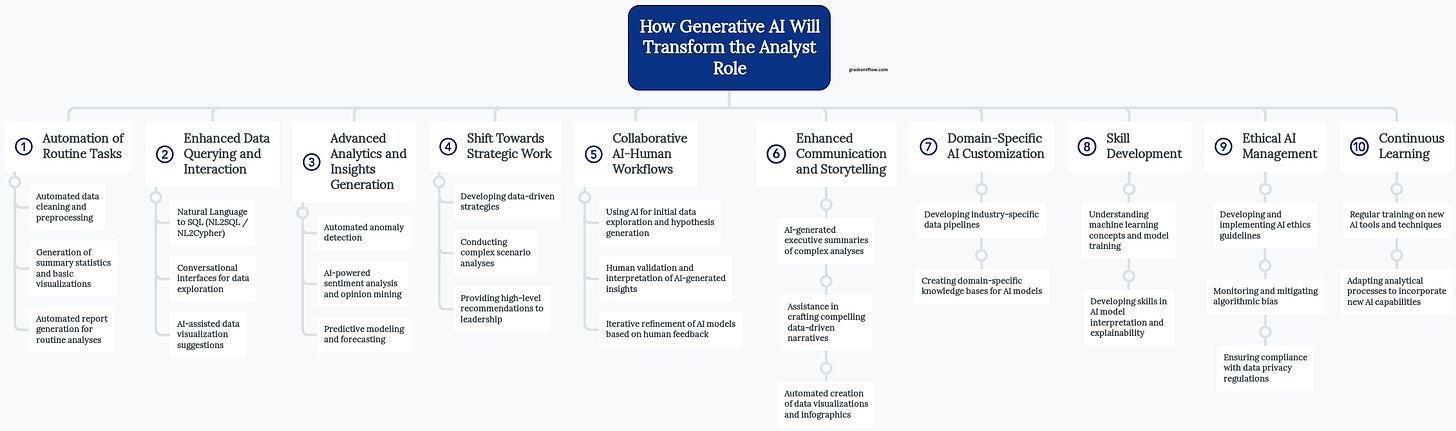
In this new landscape, the analyst will become a strategic AI orchestrator, curating high-quality data to feed and fine-tune AI models, while focusing on ethical AI management and domain-specific customization. Their unique ability to interpret AI-generated insights and align them with business objectives will make them indispensable. The real value of analysts will lie in their ability to navigate the intersection of AI tools and strategic decision-making. By leveraging both human expertise and AI, analysts will generate insights of unprecedented depth and accuracy.
The natural place to start when considering user interfaces for analysts is the familiar spreadsheet. Companies like Sigma Computing are already transforming this space by integrating Generative AI into data workflows, allowing users to ask questions, generate results, and even explain visualizations—all within a trusted and secure environment. Another interface that's beginning to incorporate Generative AI is the block-based document editor, as exemplified by Notion. Notion AI enhances productivity by automating tasks like summarizing notes, generating content, and extracting insights, all within the familiar workspace of a document editor. These developments are just the beginning, and there's significant opportunity for innovation in creating more dynamic and interactive user interfaces. Drawing inspiration from Bret Victor's ideas on dynamic user interfaces, we can envision future tools that provide immediate visual feedback, allow for direct manipulation of data, and offer contextual information. Such interfaces could dramatically enhance analysts' ability to understand complex systems, iterate quickly on ideas, and unlock new levels of creativity and productivity in their work with Generative AI. 🌟💥
I’m looking forward to Ray Summit 2024, one of my favorite conferences. See you there! The September 30-October 2 event features talks on custom AI platforms powering innovation at companies like OpenAI, Bytedance, Apple, Pinterest, Airbnb, Google, Uber, Shopify, and more. Kick things off with intensive hands-on training on September 30th, covering Ray fundamentals through advanced LLM workflows. Register with code AnyscaleBen15 to save 15% on this can't-miss AI event.
Data Exchange Podcast
Bridging the Hardware-Software Divide in AI. Features an in-depth conversation with Jay Dawani, CEO of Lemurian Labs, exploring the cutting-edge developments in AI hardware and software. The discussion covers a wide range of topics, from advanced GPU clusters and specialized AI supercomputers to software optimization and the future of AI workloads, offering insights into the challenges and innovations shaping the field of AI.
Advancing AI: Scaling, Data, Agents, Testing, and Ethical Considerations. In this episode, AI pioneer Andrew Ng delves into the challenges and opportunities in scaling deep learning models, the rise of data-centric AI, and the evolving landscape of open-source vs. proprietary AI models, while also addressing ethical concerns around AI training data and intellectual property.
AI Conference Is Next Week:
As Program Chair, I'm excited to offer this 25% discount – register now!
If you enjoyed this newsletter please support our work by encouraging your friends and colleagues to subscribe:
Ben Lorica edits the Gradient Flow newsletter. He helps organize the AI Conference, the NLP Summit, Ray Summit, and the Data+AI Summit. He is the host of the Data Exchange podcast. You can follow him on Linkedin, Twitter, Reddit, Mastodon, or TikTok. This newsletter is produced by Gradient Flow.