What We Can Learn from the FTC's OpenAI Probe
The recent investigation launched by the U.S. Federal Trade Commission (FTC) into OpenAI is a sign of the growing regulatory scrutiny of AI technology and the potential risks it poses. As we build AI models and applications, we must proactively consider the questions listed in the FTC letter. These questions address the risks of AI and outline steps that can be taken to mitigate them. The FTC’s letter highlights the importance of transparency and accountability in AI development. They encourage us to think deeply about our data sources, our training processes, and the potential risks and ethical implications of our work. By voluntarily gathering and reviewing this information, we can ensure that our models are not only technically sound but also ethically responsible and compliant with privacy standards. This proactive approach can help us build trust with our users and stakeholders, and ensure the responsible use of AI technology.
In this rapidly evolving environment, both AI technology and its regulatory landscape are in constant flux, especially in the realm of Generative AI. I consulted with my friends at Luminos.Law to assemble a list of resources, providing helpful guidance to teams and helping them understand AI-related risks and regulations.
Existing Regulations.
The EU AI Act carries significant implications for teams crafting AI systems and applications. It mandates transparency, requiring users be made aware when they're interacting with AI, including content-manipulating systems. It also tackles the use of copyrighted data in training and mandates a risk-based regulatory approach, with more stringent rules for high-risk systems. Interestingly, providers can market their systems if perceived safe, but may face sanctions if authorities disagree. The Act also bans biometric categorization, predictive policing, and software that scrapes facial images from the internet to build databases.
The Department of Consumer and Worker Protection (DCWP) in New York City has implemented a final rule concerning Automated Employment Decision Tools (AEDTs) usage by employers and employment agencies. Crucially for AI teams, the rule mandates annual bias audits for AEDTs, the results of which must be publicly disclosed. The rule specifies the requirements for bias audits, including calculating and comparing selection rates for all EEOC-reported race/ethnicity and sex categories. It stipulates the types of data permissible for audits, allows multiple employers to share a bias audit if they provide historical data, and prohibits AEDT use if its last audit is over a year old. The rule also outlines details to be included in audit summaries and provides examples when historical or test data can be used. AI teams must ensure compliance with these rules to promote fair, transparent AEDT use and avoid legal complications.
FTC Blog Posts.
The FTC has published a series of blog posts that I recommend to all businesses leveraging AI and algorithms (see [1], [2], [3]). These posts delve into essential guidelines that companies must adhere to, underlining the importance of four critical elements: transparency, accuracy, fairness, and accountability. They emphasize that deception about AI use, inaccurate data furnishing, discriminatory practices, and lack of compliance and ethics are not only unethical but can potentially lead to enforcement actions and significant fines. Designed to shed light on complex issues, these guidelines encourage responsible use of artificial intelligence.
NIST AI Risk Management Framework.
The U.S. National Institute of Standards and Technology (NIST) has published a comprehensive report on their AI Risk Management Framework (AI RMF). Key for AI teams, this guide offers in-depth information on understanding and tackling AI risks, such as risk measurement, tolerance, prioritization, and organization-wide risk management. The framework underscores the role of trustworthiness in AI systems, exploring elements like validity, safety, security, resilience, accountability, transparency, explainability, privacy, and fairness. The report details how to establish context, categorize AI systems, and document system requirements, organizational risk tolerances, and business value. This guide is an invaluable resource for AI teams to effectively understand and manage the complexities and risks involved in AI development and deployment.
FTC Letter to Open AI.
Given the increasing interest in LLMs, the FTC's questions directed at OpenAI offer key insights for teams engaged in AI modeling and applications. It's not just about acknowledging these questions; AI teams should actively prepare responses. Here are some specific elements highlighted by the FTC:
The FTC spotlights unique challenges, like model-induced "hallucinations" or the risk of inadvertently revealing personal information, which underline the necessity for strong safeguards. In terms of proactive measures, the FTC goes beyond general principles and advises on specifics, such as robust data refining and strict control over model responses, to ensure ethical AI usage.
The letter also emphasizes the importance of clear delineation of team roles and responsibilities, along with a meticulous understanding of user interactions. Addressing these specifics in the training and retraining process of LLMs can forestall the need for reactive measures down the line. By comprehending and addressing these questions, we can uphold responsible personal information handling and improve the overall reliability and safety of our AI systems.
The risks associated with Generative AI models are real and serious. Teams that are building these models need to be aware of the risks and take steps to mitigate them. The time to act is now. These risks are only going to grow in the future. Teams that want to build safe and ethical AI systems need to start taking steps today.
Unfortunately, not enough AI teams are systematically documenting the steps they are taking to manage risks associated with Generative AI models. The NIST AI Risk Management Framework is a key resource, providing a structured approach for understanding and mitigating AI risks, a perfect starting point for teams exploring AI safety and regulation.
Additionally, Luminos.Law, with their extensive experience in AI audits, can assist teams in handling sensitive issues like bias, transparency, and privacy in their AI systems. Their audits are comprehensive, efficient, and cover a wide range of AI models.
Join us at the AI Conference in San Francisco (Sep 26-27) to network with the pioneers of AI and LLM applications and learn about the latest advances in Generative AI and ML.
Data Exchange Podcast
ETL for LLMs. Brian Raymond is the founder of Unstructured, a startup building open source data pre-processing and ingestion tools specifically for Large Language Models (LLMs).
Using LLMs to Build AI Co-pilots for Knowledge Workers. Steve Hsu is a Physics Professor and co-founder of SuperFocus, a startup building LLM-backed knowledge co-pilots for enterprises.
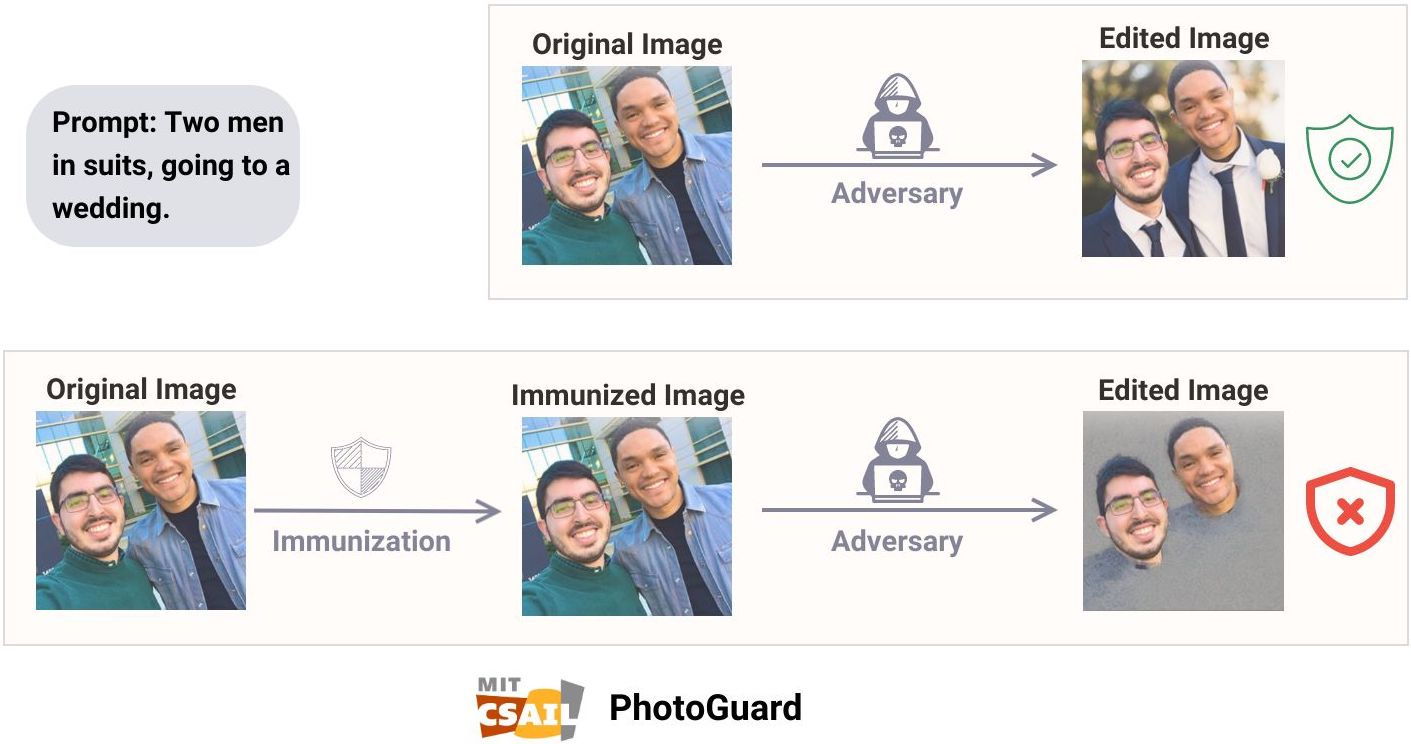
Scaling Postgres at Cloudflare
As an avid and long-term user of Postgres, I've always admired teams that operate this robust open-source database at scale. Recently, I was introduced to a small team at Cloudflare that operates a Postgres service at a truly gargantuan scale. My understanding of their work was deepened through a conversation with Vignesh Ravichandran, Engineering Manager at Cloudflare, who shared some eye-opening metrics. Vignesh and his team manage a relational database service for a powerhouse company that processes an astounding 45 million HTTP requests per second. Furthermore, among all websites utilizing a CDN, a staggering 76% rely on Cloudflare.
One thing that stood out to me was the team's significant contributions to Postgres-related software. They have made substantial modifications to PgBouncer, a lightweight connection pooler for Postgres that dramatically reduces the overhead of creating new connections. They've also worked extensively on Stolon, an open-source, cloud-native manager for high availability, a tool that ensures a database remains accessible even during system failures. These contributions, made by a lean, globally dispersed team of just five, cover everything from system building and support to guiding best practices for database usage.
The rise of vector search is being driven by the growing popularity of Generative AI and LLMs, which require efficient ways to store and search for large amounts of unstructured data. Postgres is a proven, powerful, and versatile foundation for vector search, offering AI teams scalability, security, and flexibility. pgvector is an open-source PostgreSQL extension that allows for vector similarity searches, making it a convenient option for AI teams already using Postgres. pgvector is scalable, well-documented, and already being used in production. As a testament to the vibrancy of the Postgres ecosystem, there is another option for vector search: pg_embedding is an extension that enables Hierarchical Navigable Small World (HNSW) algorithm for vector similarity search in Postgres.
Vignesh and his team continue to make their work available to the wider community. For a more detailed look into their operations, I recommend exploring their blog post and their website, which hosts key resources related to their work.
Attend the Ray Summit to learn from leading companies how they are building platforms for large-scale AI & ML applications.
If you enjoyed this newsletter please support our work by encouraging your friends and colleagues to subscribe:
Ben Lorica edits the Gradient Flow newsletter. He helps organize the AI Conference, Ray Summit, and the Data+AI Summit. He is the host of the Data Exchange podcast. You can follow him on Linkedin, or Twitter, or Mastodon, or T2, on Post. This newsletter is produced by Gradient Flow.