Avoiding the Pitfalls and Embracing the Opportunities of GenAI Adoption
By Assaf Araki and Ben Lorica.
The world of technology is no stranger to hype. From the dot-com bubble to the rise of cloud computing, we've witnessed cycles of intense excitement followed by disillusionment. Two prominent examples of transformative technologies in recent times are Big Data and Generative AI (GenAI). While Big Data promised to revolutionize decision-making through vast amounts of information, GenAI is now capturing the imagination with its ability to create content and solve complex problems. GenAI holds significant promise, but it's essential to learn from past technology hype cycles and adopt a measured approach to its implementation. By understanding the lessons from Big Data, we can navigate the GenAI landscape more effectively and ensure its responsible and beneficial adoption.
The Rise of Cloud-Native and AI-Native Products
The evolution from on-premises to cloud-native products has been transformative for many industries. Companies like Databricks, Snowflake, and Salesforce have spearheaded this shift, offering scalable and accessible solutions to organizations of all sizes. As we enter the next phase of technological advancement, AI-native products are poised to disrupt the current cloud-native landscape. Imagine a CRM application powered by GenAI, capable of not only analyzing data but also engaging customers, generating meaningful discussions, and qualifying leads for sales. The potential for AI-native solutions to revolutionize business processes is vast.
Comparing the Hype Cycles of Big Data and GenAI
The Big Data hype cycle followed a familiar trajectory: initial euphoria and grand promises of a data-driven revolution, followed by the harsh reality of unfulfilled expectations, disillusionment, and underwhelming results. Today, GenAI is riding a similar wave of hype, propelled by management pressure and a fear of missing out. This enthusiasm, while understandable, risks overlooking the technology's limitations and fostering unrealistic expectations. Such miscalculations can lead to misaligned strategies and suboptimal implementations, ultimately hindering the potential benefits of GenAI.
Common Pitfalls and Lessons Learned
A critical misstep in adopting both Big Data and GenAI is the disconnect between business needs and technology. Organizations often rush to embrace these technologies without clearly defining the problems they aim to solve or assessing the potential value they offer. They operate in a bottom-up approach, rushing to build Hadoop clusters and integrate all the data in a data lake instead of working top-down to solve a business use case that leverages big data and utilizes a platform. Users often miss the fact that technologies like Big Data and GenAI are not the end goal but tools to build products. This misalignment can lead to wasted resources and disillusionment.
GenAI shows promise, but we must learn from past hype and adopt a measured approach
Another challenge lies in the talent and infrastructure gap. While Big Data demanded significant platform investments, GenAI can leverage existing services and models, potentially lowering the entry barrier. Initially, Big Data faced the talent challenge of users adopting new domain dpecific languages (DSLs). Encapsulating the MapReduce DSL with SQL opened the door to a large and mature community that can use Big Data. GenAI hasn't yet developed its DSL; building one and proliferating it among the community will take time. However, the need for specialized talent to implement and manage GenAI solutions remains a hurdle.
Furthermore, integration and data management issues plagued many Big Data initiatives, resulting in data swamps and underutilized resources. GenAI faces similar risks if not seamlessly integrated into the organizational fabric and data ecosystem.
GenAI Applications Beyond the Hype
While GenAI's creative capabilities generate significant buzz, its potential extends far beyond content generation. GenAI can revolutionize traditional AI tasks like entity extraction, sentiment analysis, and summarization. By fine-tuning pre-trained language models, GenAI can surpass the performance of proprietary models while simultaneously reducing development and maintenance costs. Imagine an RPA application powered by GenAI, replacing a proprietary model that requires constant attention from data scientists. This GenAI-powered application could seamlessly extract entities, relations, sentiment, and other data points from semi-structured documents and automatically populate the ERP system. This presents a compelling opportunity for startups and established enterprises alike to automate expensive operational processes and minimize go-to-market risks. By leveraging GenAI for both novel and established AI applications, organizations can unlock significant efficiency gains and cost savings.
Assessing the Feasibility of AI Adoption
A recent MIT paper sheds light on the nuanced reality of AI adoption, emphasizing that its integration will be more gradual than initially anticipated. The paper underscores the importance of selective automation, focusing on tasks that are both technically feasible and economically viable. For Data and AI teams, this translates to conducting thorough feasibility assessments that encompass technical capabilities, cost-benefit analysis, and alignment with organizational goals. Notably, the study reveals that only 23% of worker wages associated with vision tasks currently present an economically attractive opportunity for automation. This finding underscores the need for a phased and iterative approach to AI implementation, allowing organizations to adapt to evolving technologies and cost dynamics while maximizing the value of their AI investments.
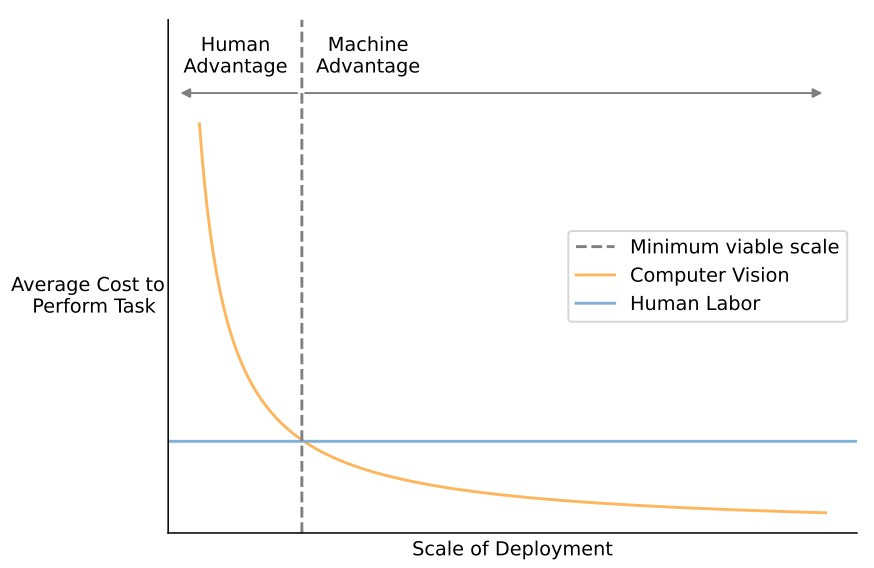
Navigating the GenAI Landscape: Challenges and Strategies
Quantifying the return on investment (ROI) for GenAI initiatives remains a complex task, with organizations often relying on proxy metrics like productivity gains and customer satisfaction. As the technology matures, the focus will shift towards more concrete valuation methods that directly measure revenue generation, cost savings, and efficiency improvements. Implementing and scaling GenAI also presents a challenge due to the scarcity of specialized talent within many enterprises. While model providers currently offer professional services to bridge this gap, developing in-house expertise will be essential for long-term success and competitive advantage. Interestingly, while cost is a factor in GenAI adoption, control over data and the ability to customize models are the primary drivers for embracing open-source solutions. Recognizing the rapidly evolving nature of the GenAI market, enterprises are also prioritizing flexibility, designing applications that can seamlessly switch between different models as needed.
Learning from the Past: Avoiding Big Data Pitfalls in the GenAI Era
To avoid the mistakes of the Big Data era, organizations must clearly define business problems and use cases before adopting GenAI. Building a data-driven culture and addressing employee concerns are critical for successful implementation. Proper data integration and infrastructure modernization are also essential to avoid data silos and underutilization. Investing in skilled talent and promoting diversity in the field will be key to unlocking the full potential of GenAI. Prioritizing data quality, governance, and ethical usage will help mitigate risks and ensure responsible adoption. Finally, embracing an incremental and iterative implementation approach allows for course corrections and adaptations as the technology continues to evolve. By adhering to these principles, organizations can harness the transformative power of GenAI while avoiding the missteps of the past.
By following these principles, organizations can leverage GenAI's benefits while avoiding past mistakes
Democratizing GenAI
Generative AI (GenAI) holds immense potential, but the path to adoption is often paved with challenges. Resource constraints and a need for more skilled experts can make it difficult for businesses to fully embrace data analytics and AI. To bridge this gap and empower organizations, solution providers should prioritize developing affordable, user-friendly solutions that deliver tangible benefits and demonstrate quick wins.
Businesses should start by leveraging APIs, endpoints, and GenAI Software as a Service (SaaS) to bridge the gap between orchestration and fine-tuning before investing in building their own platform. They must reach a critical mass of GenAI applications before transitioning to a single-tenant GenAI platform. By forging strategic partnerships, providing tailored training programs, and helping small and medium-sized enterprises (SMEs) leverage open data sources, AI teams can equip these businesses with the tools and knowledge they need to overcome resource limitations and drive innovation within their respective industries.
Conclusion
As we navigate the current wave of excitement surrounding GenAI, it's imperative to learn from the missteps of the Big Data era. By adopting a deliberate and strategic approach, organizations can chart a path to successful GenAI implementation and unlock its transformative potential. This includes clearly defining business problems, fostering a data-driven culture, ensuring seamless data integration, investing in skilled talent, prioritizing data quality and governance, and embracing an incremental and iterative approach. While the emergence of AI-native products presents a significant opportunity for disruption and innovation, it must be approached with both ambition and responsibility. Data and AI teams have a crucial role to play in guiding their organizations through this journey, leveraging the lessons of the past to shape a future where GenAI technologies are implemented ethically, effectively, and for the benefit of all.
Data Exchange Podcast
DBRX and the Future of Open LLMs. Hagay Lupesko from Databricks delves into the development of DBRX, an open LLM that aims to deliver high-quality AI performance at a cost-effective price point. By leveraging innovative techniques and the power of the open-source community, DBRX represents a step forward in making advanced AI more accessible and adaptable for various applications.
2024 Artificial Intelligence Index. We explore advancements in AI with Nestor Maslej, editor of the 2024 Artificial Intelligence Index Report from Stanford's Institute for Human-Centered Artificial Intelligence. The conversation highlights the evolving landscape of AI benchmarking and the importance of adapting metrics to reflect real-world applications and human evaluation in AI development.
If you enjoyed this newsletter please support our work by encouraging your friends and colleagues to subscribe:
Ben Lorica edits the Gradient Flow newsletter. He helps organize the AI Conference, Ray Summit, and the Data+AI Summit. He is the host of the Data Exchange podcast. You can follow him on Linkedin, Twitter, Reddit, or Mastodon. This newsletter is produced by Gradient Flow.