Generative AI in Finance: Opportunities & Challenges
The Financial Services Sector's March into Generative AI
During my time as a quant at a hedge fund, I was struck by the asset management industry's willingness to adopt cutting-edge technologies. Hedge funds are constantly looking for new ways to gain an edge in the increasingly competitive financial markets. They are quick to invest in and experiment with novel technologies and data streams in the hopes of finding a competitive edge.
As the world shifts towards an AI-driven future, the Financial Services and Banking sector (FSB) is once again at the forefront of innovation. I recently examined the largest companies in this space and detected strong interest in Generative AI (GAI) and Large Language Models (LLMs). Recent job postings indicate that outside the technology domain, the most pronounced demand for GAI expertise is rooted in the FSB industry:
These technologies have the potential to transform the way the FSB sector operates, providing new opportunities for efficiency, personalization, and risk management. LLMs can efficiently process large amounts of unstructured data and provide deeper insights into market dynamics. This is just the tip of the iceberg, as GAI and LLMs can be used for a wide range of use cases, from predictive analytics and real-time insights to automation in reporting.
Generative AI Use Cases
Not many GAI and LLM solutions are all tailored to meet the stringent requirements of the financial services industry. It's also worth noting that many GAI and LLM startups I’ve encountered lean towards the creation of general-purpose tools. However, as our discussions on Pegacorns have highlighted, startups that target specific domains and applications often attain greater success and relevance. Domain specificity is crucial, especially in an intricate sector like the FSB.
Despite the interest in GAI and LLM technologies in the FSB sector, keep in mind that most projects are still in the early stages of development. GAI and LLMs are complex technologies that necessitate specialized expertise for their safe development and deployment, especially in finance where the applications often have stringent requirements. Additionally, the FSB sector is heavily regulated, which adds an additional layer of complexity to any AI project. Using the example of a recent article where I explored the specialized system of entity resolution, it becomes evident how swiftly AI undertakings can escalate in complexity. Both entity resolution and master data management, which merge big data with real-time processing and AI-driven strategies, highlight the intricate nature of modern enterprise AI applications.
Nonetheless, GAI and LLMs are beginning to enable novel prototypes across a wide range of functions. At the heart of this potential revolution is the capability to process and unlock vast swathes of data. For instance, financial powerhouses like Goldman Sachs have harnessed AI's power to efficiently extract and categorize data from a multitude of documents. Meanwhile, Citigroup delves into the nuances of unstructured data—from emails to intricate legal documents—seeking out valuable insights and patterns.
Process automation, too, has undergone a significant metamorphosis. Citigroup, for example, is beginning to use Generative AI for tasks as complex as code writing and desk-based research. No longer confined to the domain of the tech-savvy, non-technical employees at institutions such as Goldman Sachs can now automate tasks, with LLMs serving as a potent tool.
These tools go beyond simple data management and process improvement. They can also potentially transform vital areas of risk and fraud management. Wells Fargo is beginning to use these technologies for robust risk assessment and detecting fraudulent activities.
Customer and investor relations, often the lifeblood of financial institutions, won’t be left untouched either. AI-driven initiatives are enhancing customer service and facilitating the generation of investment opinions. Firms like J.P Morgan and Truist Financial Corp are exploring the vast potential of LLMs in understanding and catering to their clientele better. Additionally, American Express is using AI for product personalization, ensuring an ever-evolving, customer-centric approach.
Beyond these, the purview of Generative AI in the FSB sector stretches into compliance, market analysis, and even strategy formulation. Whether it's upholding rigorous regulatory standards or crafting insightful investment strategies, companies such as Wells Fargo and American Express are starting to demonstrate the practical integration of Generative AI in finance.
Key Challenges
The potential of Generative AI and LLMs to enhance and transform the financial landscape is immense, especially as these applications become capable of scaling to a large user base, rapidly process vast torrents of real-time data, and deliver prompt, reliable responses.
However, the journey of integrating these innovations into the FSB sector isn't without its hurdles. As one of the most heavily regulated industries, the Financial Services sector needs more than just groundbreaking technology; it demands assurance, compliance, and meticulous attention to detail. This raises a spectrum of challenges, from training models to make financially sound decisions, to grappling with issues like job displacement and the evolving landscape of patenting AI tools. These aren’t mere technical problems; they represent significant socio-economic, legal, and operational dimensions.
Summary
In conclusion, Generative AI and Large Language Models are drawing strong interest within the Financial Services and Banking sector. These technologies can potentially improve efficiency, personalization, and risk management in a variety of ways. However, there are also challenges to the adoption of GAI and LLMs in the FSB sector, such as the need for domain specific models, regulatory compliance, and the potential for job displacement. By focusing on addressing the specific challenges of the financial services sector, startups and vendors can help to pave the way for the widespread adoption of GAI and LLMs in this industry.
Join us at the AI Conference in San Francisco (Sep 26-27) to network with the pioneers of AI and LLM applications and learn about the latest advances in Generative AI and ML.
Data Exchange Podcast
Exploring the Future of Software Development with AI and LLMs. Michele Catasta is VP of AI at Replit, an AI-powered software development platform that allows teams to build and deploy applications on any device, without any setup required.
A Lightweight SDK for Integrating AI Models and Plugins. Alex Chao is a Product Manager at Microsoft focused on Semantic Kernel, an open-source AI and LLM orchestrator.
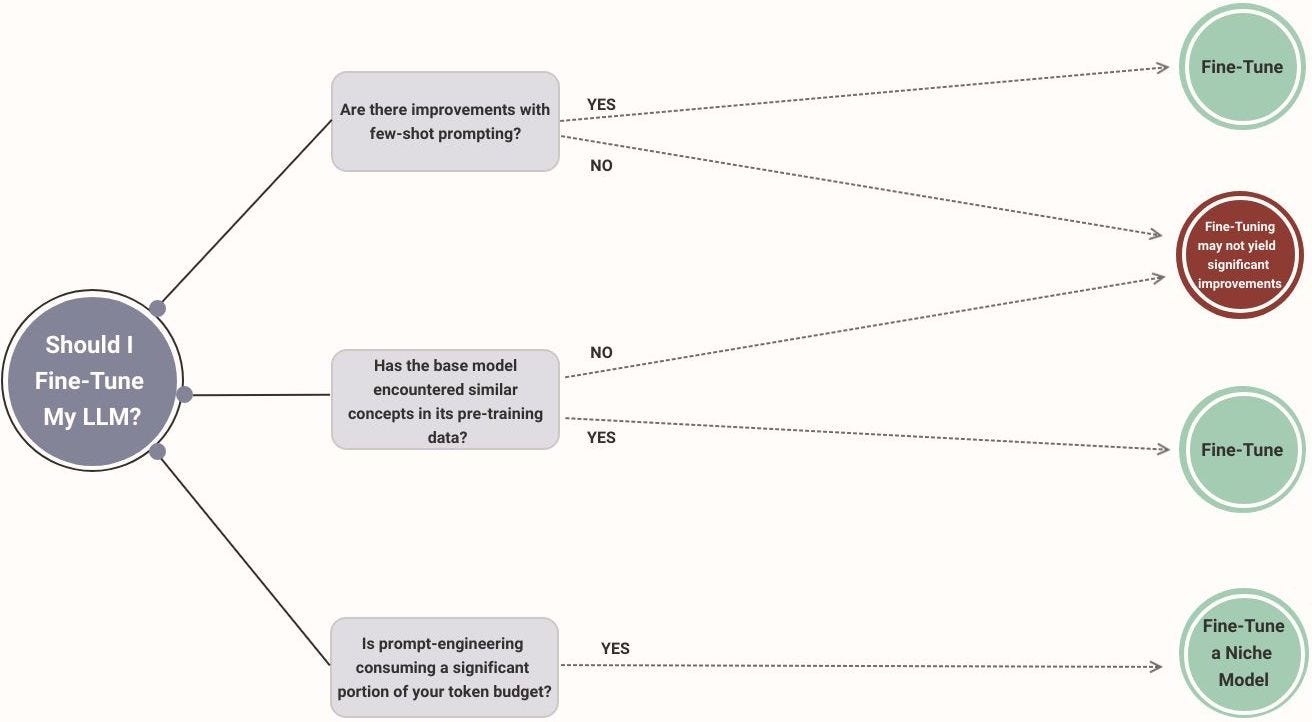
Llama's Emerging Ecosystem
Llama was Meta AI's most performant LLM for researchers and non-commercial use cases. It was more parameter-efficient than other large commercial LLMs, meaning that it could achieve comparable performance to larger models with fewer parameters. In fact, Llama outperformed GPT-3 on many benchmarks, despite being smaller.
Llama 2 is the successor to Llama and has several improvements over its predecessor. It was trained on 2 trillion tokens of data from publicly available sources, which is 40% more than Llama. It also has a context length of 4096 tokens, which is twice the context length of Llama. These improvements allow Llama 2 to better understand and generate text in more complex and nuanced ways.
An important aspect of Llama 2 is its license, which permits commercial use. This means that startups, researchers, and LLM enthusiasts can build Llama 2 into commercial applications without much concern for licensing issues. This has led to a proliferation of tools and resources related to Llama, including
Instructions and guides on how to deploy and operate the Llama model on local machines and environments. This makes it possible for anyone to experiment with Llama and see how it can be used for their own projects.
Resources discussing code implementations, porting, and other development-related tasks associated with Llama. This helps developers to understand how Llama works and to make it more compatible with their own applications.
Customization and fine-tuning: by adjusting the model to specific applications, users can achieve remarkable success rates, a testament to Llama's versatility.
LLama has inspired projects like llama.cpp, an open source tool that provides C++ inference for the LLaMa model. It is self-contained and has no dependencies, making it easy to deploy. This versatility means that llama.cpp can be used to integrate LLaMa into a wide range of applications and environments, from laptops to mobile devices.
There are several resources focused on fine-tuning Llama, including a recent detailed guide from Anyscale. This must-read article critically examines the potential of fine-tuning models to surpass other methods like prompt engineering for specific tasks, taking into account factors such as new concepts, the effectiveness of few-shot prompting, and token budget constraints. It also discusses the evaluation process in detail, providing a clear roadmap for teams to follow.
Beyond the technical aspects, there is a growing set of tools designed explicitly for Llama. Innovations such as a custom summary system that interacts with ChatGPT have emerged, enhancing the user's ability to extract and interact with past conversation data. Global tech giants like Amazon, Baidu and Alibaba are also on the Llama bandwagon, offering it as a service. This movement amplifies Llama's reach and applicability, as users can integrate it with private applications using proprietary data sets. But perhaps the most compelling testament to the Llama ecosystem is the real-world applications that are sprouting. Collaborations like that between Meta and Qualcomm are opening avenues for embedding advanced AI models in everyday mobile devices, profoundly influencing user experiences.
In conclusion, open source models are likely to continue to drive innovation in Generative AI. This is because they allow researchers and developers to experiment with different models and techniques, share their findings with the wider community, and build products that rely on these models. Other LLMs and foundation models will likely inspire similar activity if they show decent baseline performance and have the right open source license.
Use the discount code GradientFlow to attend Ray Summit and discover firsthand how leading companies are building Generative AI & ML applications and platforms.
If you enjoyed this newsletter please support our work by encouraging your friends and colleagues to subscribe:
Ben Lorica edits the Gradient Flow newsletter. He helps organize the AI Conference, Ray Summit, and the Data+AI Summit. He is the host of the Data Exchange podcast. You can follow him on Linkedin, or Twitter, or Mastodon, or T2, on Post. This newsletter is produced by Gradient Flow.